Understanding Labeling Tools for Machine Learning
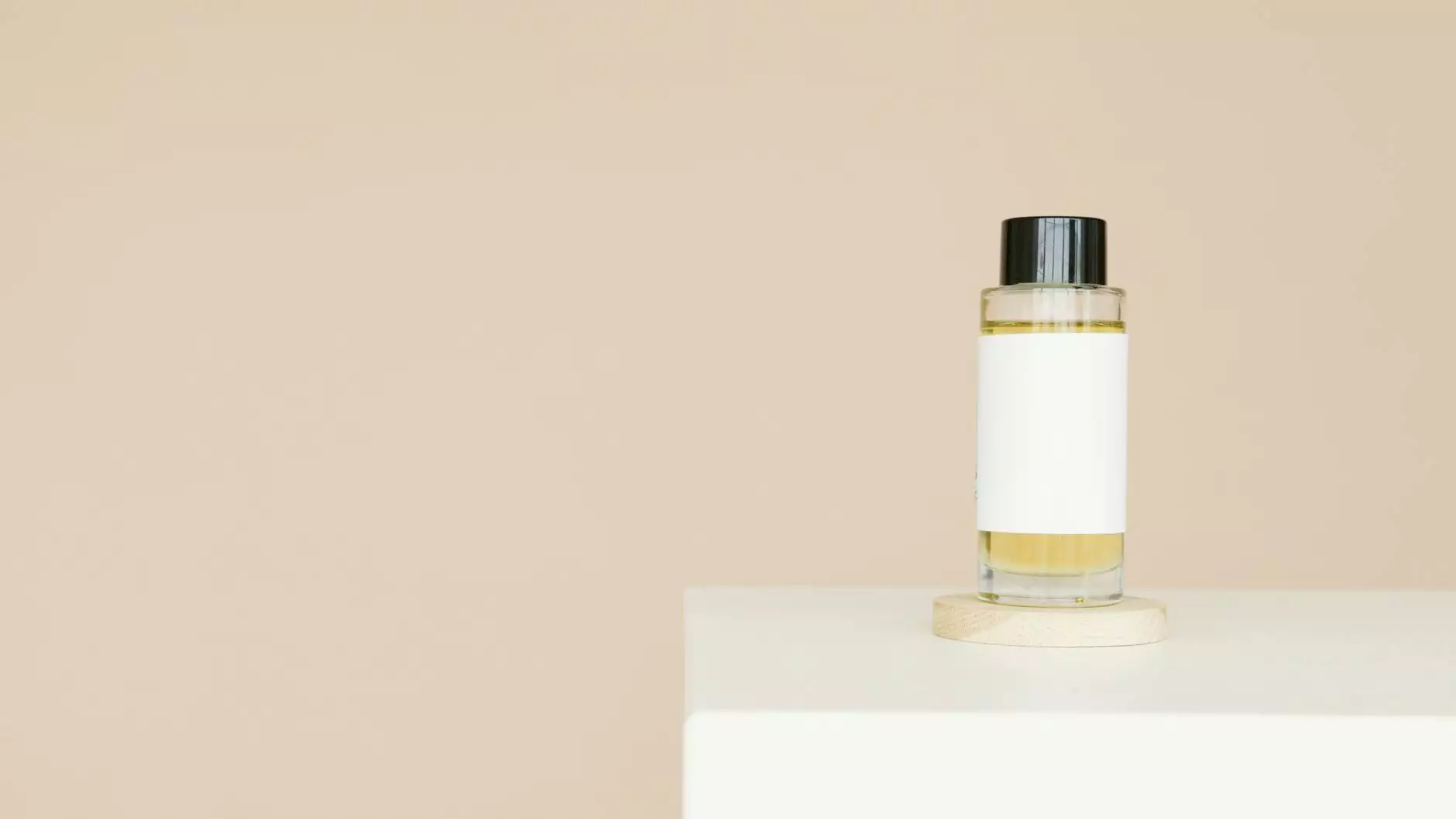
In the rapidly advancing world of artificial intelligence and machine learning, leveraging quality data is paramount. One of the foundational steps in any machine learning project is the effective labeling of data. This is where labeling tools for machine learning come into play. These tools help automate and streamline the data annotation process, ensuring that algorithms have the correct input to learn from.
What Are Labeling Tools?
Labeling tools are specialized software applications designed to annotate datasets, preparing them for training machine learning models. Annotation can include tasks such as:
- Image Tagging: Identifying and labeling objects within images.
- Text Annotation: Assigning categories, sentiments, or entities to text data.
- Audio and Video Annotation: Marking specific events or attributes in sound or visual media.
These tools enable companies to build rich datasets that enhance model performance and accuracy.
The Importance of Data Annotation in Machine Learning
To understand why labeling tools are essential, we need to consider the concept of data annotation itself. Data annotation is the process of tagging data to enable machine learning algorithms to understand the input, thereby creating a structure within unstructured data. Here’s why this is crucial:
- Model Accuracy: High-quality labeled data significantly improves the accuracy of machine learning models.
- Model Training: Labeled data is used to train models, which generates reliable predictions and classifications.
- Automation Capability: Properly labeled data allows for better automation of decision-making processes.
- Market Competitiveness: Accurate models give businesses an edge through improved data-driven decisions.
Key Features of Effective Labeling Tools
When choosing labeling tools for machine learning, there are several key features to consider:
- Scalability: The tool should handle large volumes of data as your business grows.
- User-Friendliness: A simple interface reduces the learning curve and increases productivity.
- Collaboration Tools: Effective tools allow teams to work together seamlessly, managing tasks and monitoring progress.
- Quality Control: Features that ensure data accuracy through reviews and validation are crucial.
- Integration: The ability to integrate with other software solutions assists in creating a smooth workflow.
Types of Labeling Tools for Machine Learning
Labeling tools can be categorized into various types based on their functions and applications:
- Manual Annotation Tools: These require human input for tagging data. Examples include KeyLabs which provides an intuitive interface for manual data annotation tasks.
- Automated Annotation Tools: These use algorithms to suggest labels, greatly speeding up the process while still requiring human oversight.
- Hybrid Tools: Combinations of both manual and automated approaches, these tools offer flexibility in the annotation process.
Integrating Labeling Tools in Your Workflow
To ensure that your machine learning projects achieve optimal results, it is essential to integrate labeling tools effectively into your existing workflow. Here are steps to consider:
- Evaluate Your Needs: Assess the scale and complexity of the data you will be working with.
- Select the Right Tools: Choose labeling tools that align with your specific requirements and technical capabilities.
- Train Your Team: Invest time in training your team to use the tools to maximize efficiency.
- Measure and Analyze: Track the effectiveness of the tools through metrics like annotation speed, quality, and impact on model performance.
Benefits of Using KeyLabs for Data Annotation
One of the leading platforms in this space is KeyLabs. Here’s why it stands out:
- Enterprise-Grade Solution: KeyLabs offers a robust platform tailored for enterprises looking for scalable data annotation.
- Versatile Annotation Options: Support for various data types including text, image, and audio makes it suitable for diverse projects.
- Advanced Quality Assurance: Seamless quality control processes ensure data integrity and accuracy.
- Collaborative Environment: Facilitate team collaboration with integrated tools that keep everyone on the same page.
- Custom Workflow Capabilities: You can easily adapt the platform to fit your specific annotation process.
Challenges in Data Annotation and How to Overcome Them
Despite the many advantages of labeling tools, there are challenges developers and businesses face, including:
- High Labor Costs: Data annotation can be labor-intensive. Automation features of modern tools can alleviate this issue.
- Data Privacy Concerns: Ensuring data compliance is vital. Choose tools with robust security measures in place.
- Data Quality Issues: Poor-quality annotations can lead to ineffective models. Regular monitoring and quality checks are necessary.
By understanding these challenges and utilizing KeyLabs’ advanced features, businesses can effectively manage the annotation process and produce high-quality data.
The Future of Labeling Tools in Machine Learning
The landscape of machine learning is constantly evolving, and so are the tools available for data annotation. Innovations in artificial intelligence are leading to more powerful labeling tools that not only increase speed and efficiency but also improve the accuracy of annotations. Future trends may include:
- AI-Assisted Annotation: Tools that leverage AI to enhance the labeling process.
- Predictive Annotation: Systems that can pre-label data based on historical contexts.
- Enhanced User Interfaces: More intuitive interfaces that allow even non-technical users to engage in data annotation.
Conclusion: The Importance of Choosing the Right Labeling Tools
In conclusion, the choice of labeling tools for machine learning can significantly impact the success of your data-driven projects. By investing in robust annotation tools like those offered by KeyLabs, businesses can enhance their data quality, improve model performance, and maintain a competitive edge. Proper data annotation is not just a necessity; it is an opportunity to unlock the full potential of your data.