Revolutionizing Business Efficiency Through Data Labeling
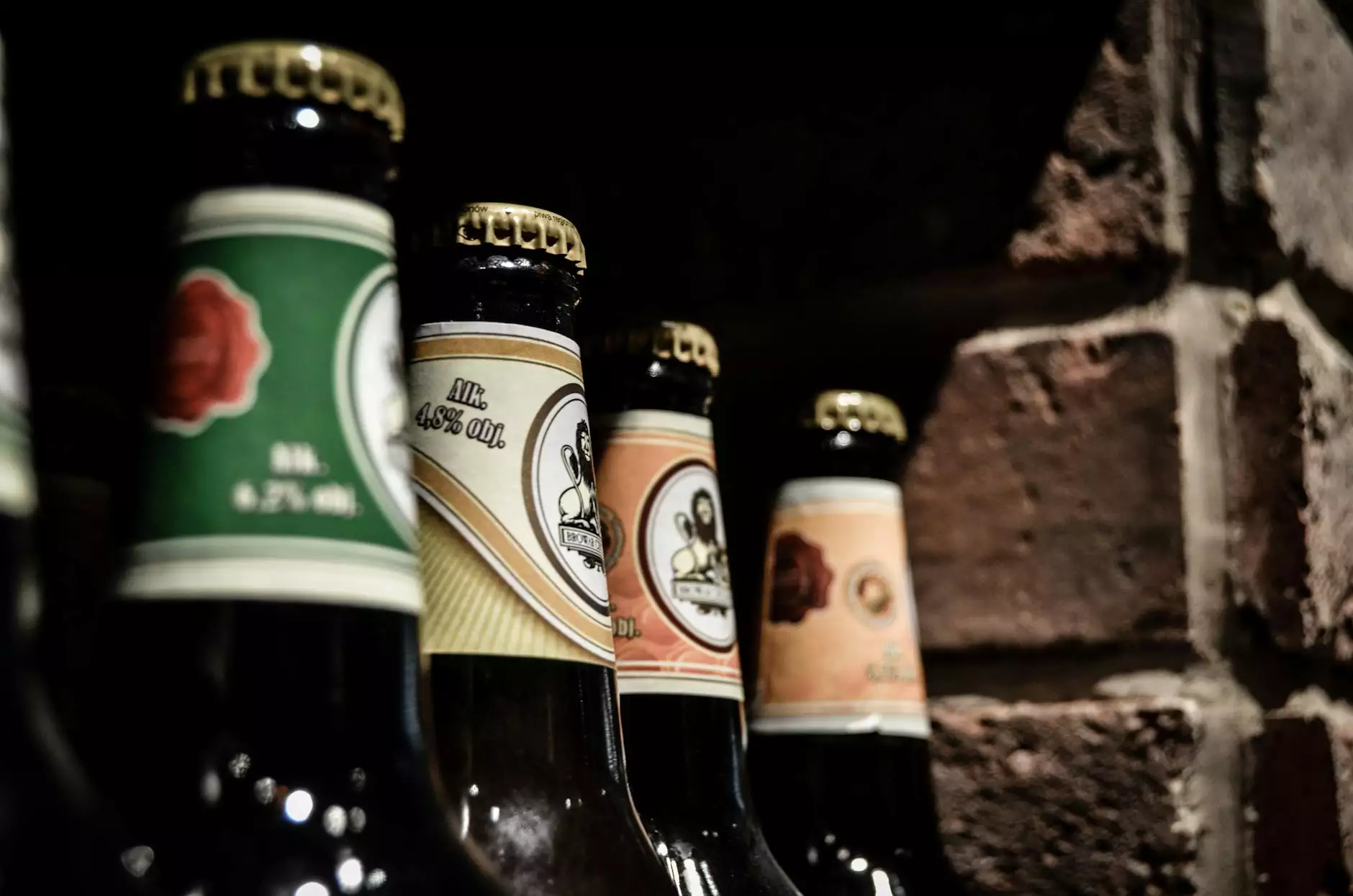
In an era driven by technology and data, businesses are continually seeking innovative solutions to improve their processes and outcomes. Among the many methods that have emerged to enhance operational efficiency is data labeling. This practice is essential for developing machine learning models and artificial intelligence systems that require accurately annotated datasets to function effectively. In this comprehensive article, we will delve into the significance of data labeling, the tools available for effective annotation, and how businesses can leverage these technologies to stay ahead in today's competitive landscape.
Understanding the Importance of Data Labeling
Data labeling is the process of tagging or annotating data, allowing machines to understand it. This foundational step is crucial in training algorithms for various applications, from image recognition to natural language processing. Here are some vital points regarding the importance of data labeling:
- Enhances AI Accuracy: Properly labeled data ensures that machine learning models can make the most accurate predictions. The quality of your results directly correlates with the quality of your data.
- Improves Decision Making: Businesses that utilize labeled data can derive insights that lead to better strategic decisions. These insights can affect everything from marketing strategies to product development.
- Drives Automation: Automated systems require well-labeled datasets to function efficiently. Data labeling paves the way for companies to embrace automation and streamline their operations.
- Facilitates Compliance: In regulated industries, proper data annotation aids in maintaining compliance with data governance and privacy regulations.
Types of Data Labeling Techniques
There are several methods of data labeling, each suited to different contexts and datasets. Here, we will explore some of the most common techniques:
1. Manual Labeling
In manual labeling, human annotators review and tag data based on predefined guidelines. While this method is accurate, it can be time-consuming and may introduce human error. However, manual labeling is essential for complex datasets requiring nuanced understanding, such as sentiment analysis in text data.
2. Semi-Automatic Labeling
This technique combines automation with human oversight. Initial labeling is conducted by automated tools, while human annotators refine and correct the labels. This hybrid method increases efficiency while maintaining a high degree of accuracy.
3. Automatic Labeling
Using machine learning and AI, automatic labeling systems can rapidly annotate large datasets. This method is particularly useful for large-scale projects but requires high-quality training data to function effectively.
The Role of Data Annotation Tools and Platforms
The success of data labeling greatly depends on the tools and platforms utilized. Businesses can significantly enhance data labeling efficiency by employing robust annotation tools. Here are some standout features to consider in a data annotation platform:
- User-Friendly Interface: A platform with an intuitive interface allows annotators to work quickly and effectively, reducing the learning curve associated with new software.
- Collaboration Features: Team collaboration tools help multiple annotators work on the same projects, enhancing productivity and ensuring consistency in labeling standards.
- Quality Control Mechanisms: Annotation platforms that offer built-in quality checks ensure high accuracy in data labeling through peer reviews and automated audits.
- Scalability: Efficient platforms can easily adapt to changing datasets and business needs, allowing organizations to scale their operations without compromising quality.
How Data Labeling Influences Various Industries
The impact of data labeling transcends multiple sectors, facilitating innovation and streamlining operations across different fields:
1. Healthcare
In healthcare, properly labeled data is vital for training models that assist in diagnosis, patient monitoring, and treatment recommendations. By employing advanced data annotation tools, healthcare organizations can improve patient outcomes and operational efficiency.
2. Automotive
The automotive industry is leveraging data labeling for the development of autonomous vehicles. Annotated datasets help in training models that interpret sensor data, enabling high-accuracy in navigation and safety features.
3. Retail
Retailers use data labeling to analyze customer behavior through sentiment analysis, recommendation systems, and inventory management. Accurate labeling allows businesses to better understand market trends and consumer preferences.
4. Finance
In finance, data labeling plays a crucial role in fraud detection, algorithmic trading, and risk assessment. High-quality labeled data is essential for developing models that protect against financial crimes and optimize investment strategies.
Best Practices for Effective Data Labeling
To achieve the best outcomes from data labeling, organizations should follow certain best practices to maximize quality and efficiency:
- Define Clear Guidelines: Establishing well-defined labeling rules and guidelines ensures that all annotators are on the same page, leading to consistent and high-quality outputs.
- Choose the Right Tool: Select annotation tools that fit your specific needs, enabling flexibility and efficiency throughout the labeling process.
- Conduct Regular Training: Providing training for annotators can enhance their skills and improve the overall quality of the labeled data.
- Implement Quality Assurance: Regularly audit labeled data to ensure accuracy and consistency, facilitating continuous improvement.
The Future of Data Labeling
The landscape of data labeling is continuously evolving, influenced by advancements in technology and increasing demands for data-driven decision-making. Here are some emerging trends:
1. AI-Driven Annotation
As machine learning models become more sophisticated, they are increasingly capable of automating the labeling process. AI-driven annotation tools are set to revolutionize how data is labeled, requiring less human intervention.
2. Crowdsourcing in Data Labeling
Crowdsourcing is becoming a popular method of data labeling, allowing businesses to tap into a vast pool of annotators. This approach can significantly reduce costs and expedite the labeling process.
3. Enhanced Collaboration Tools
Future data annotation platforms will likely integrate more advanced collaboration features, promoting teamwork and improving the quality control process.
Conclusion
The importance of data labeling cannot be overstated, as it serves as a critical foundation for machine learning and AI applications across various industries. By leveraging sophisticated data annotation tools and following best practices, organizations can gain a competitive edge in their respective markets. Keylabs.ai is at the forefront of this revolution, offering cutting-edge solutions that facilitate effective data labeling and annotation. Embracing these innovations will empower businesses to harness the full potential of their data, driving growth and success in today's dynamic environment.