Unlocking the Power of Image Datasets for Classification in Business
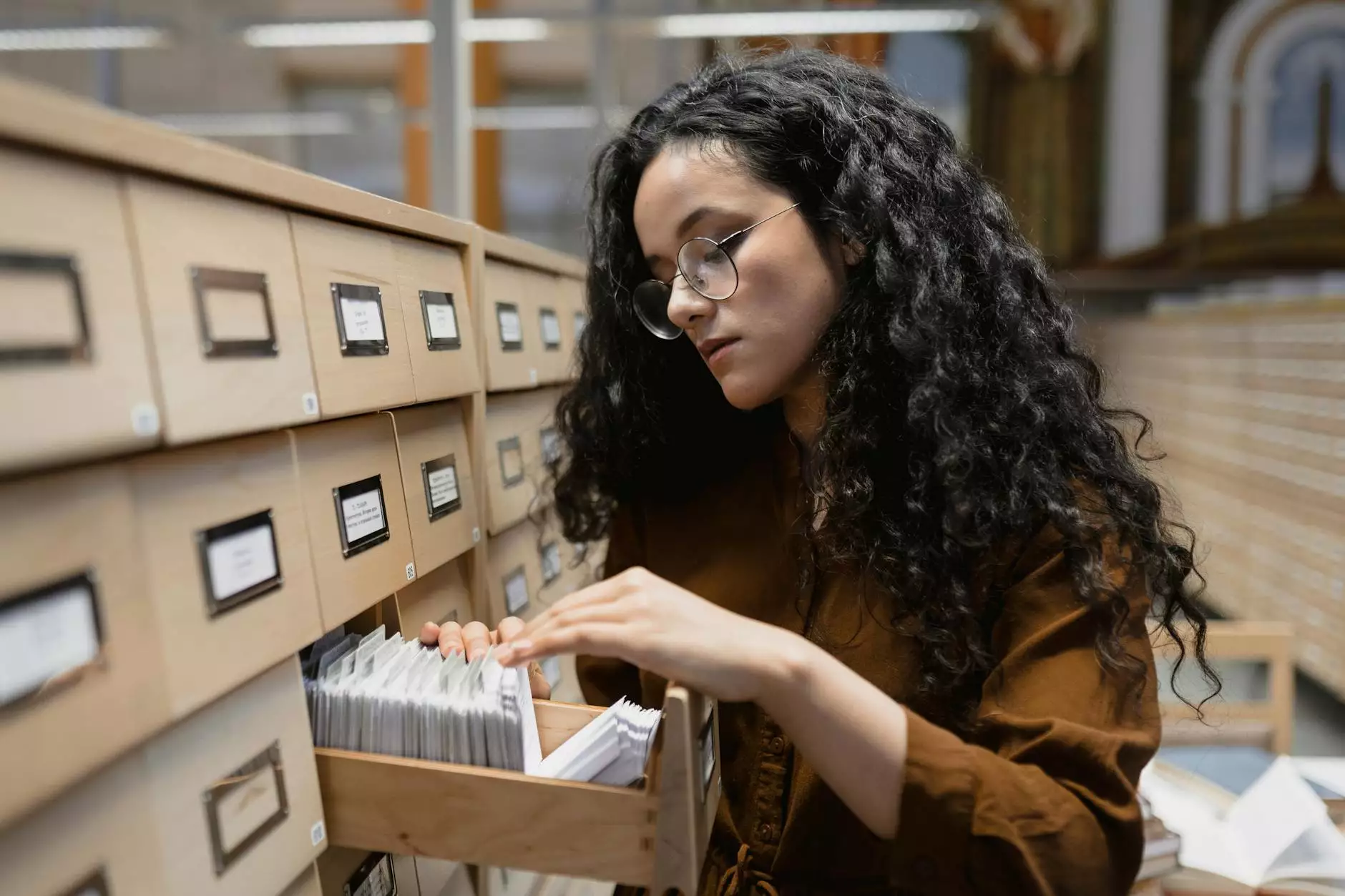
In recent years, the digital transformation of businesses has accelerated, and one of the driving forces behind this revolution is the utilization of image datasets for classification. As companies leverage machine learning (ML) and artificial intelligence (AI) technologies, the importance of high-quality annotated data has never been greater. In this article, we will explore how image datasets can enhance business operations, the role of data annotation tools, and why investing in a robust data annotation platform is essential for future success.
The Significance of Image Datasets in Business
Businesses across various sectors are increasingly adopting AI-driven solutions to optimize their operations, improve customer experiences, and gain a competitive edge. Central to these AI applications is the concept of image datasets for classification, which involves using annotated images to train machine learning models to recognize patterns, objects, and features within images.
Key Benefits of Image Classification
- Enhanced Decision Making: By leveraging image classification, businesses can make data-driven decisions based on visual data analysis.
- Improved Customer Experience: Utilizing AI for image recognition can enhance customer interactions, enabling personalized recommendations and services.
- Increased Operational Efficiency: Automating image-related tasks can streamline processes, reducing the need for manual input and minimizing errors.
- Cost Savings: Effective image classification can lead to significant reductions in operational costs by minimizing waste and inefficiencies.
Understanding Data Annotation for Image Classification
Data annotation is the process of labeling or tagging data to make it understandable for machines. For image datasets for classification, annotation involves identifying and marking relevant features within images, allowing algorithms to learn from the annotated data. High-quality annotated data is critical for the accuracy and effectiveness of machine learning models.
Types of Image Annotation Methods
There are several methods of image annotation used for classification purposes:
- Bounding Boxes: A rectangular box around a detected object, commonly used in object detection.
- Polygon Annotation: More precise than bounding boxes, this method outlines complex shapes or objects within an image.
- Semantic Segmentation: This involves dividing an image into segments, where each pixel is classified, suitable for applications like autonomous driving.
- Image Classification: Labels are assigned to entire images, suitable for recognizing overall themes or categories.
Selecting the Right Data Annotation Tool
To ensure the accuracy and quality of your image datasets for classification, choosing the right data annotation tool is crucial. A robust tool should offer a range of features tailored to your business needs, including:
Features to Look for in Data Annotation Tools
- User-Friendly Interface: An intuitive platform that requires minimal training facilitates quicker project turnarounds.
- Supports Multiple Annotation Types: Flexibility in annotation types helps in catering to various ML project needs.
- Collaboration Features: Enables teams to work together efficiently in real time, enhancing productivity.
- Quality Assurance Mechanisms: Systems to review and validate annotations minimize human errors.
- Integration Capabilities: The tool should easily integrate with existing technology stacks and workflows.
Data Annotation Platforms: A Game Changer for Businesses
Investing in a comprehensive data annotation platform can significantly impact how businesses handle their image datasets for classification. These platforms bring together various tools and resources, streamlining the annotation process and offering scalability as your needs evolve.
Benefits of Data Annotation Platforms
- Scalability: As businesses grow, their data annotation needs change. A platform allows for scaling up or down based on demand.
- Cost-Effectiveness: Centralized tools often reduce costs associated with managing multiple disparate systems.
- Access to Expert Annotators: Many platforms provide access to teams of trained annotators who ensure high-quality outputs.
- Advanced Technologies: Features such as AI-assisted annotation can significantly speed up the process while maintaining accuracy.
Implementation Strategies for Image Classification Projects
Once you have chosen your data annotation tools and platforms, it's important to develop a strategic approach to implementing your image datasets for classification projects. Here are some steps to consider:
Steps for Successful Implementation
- Define Your Objectives: Clearly outline what you hope to achieve through image classification.
- Create a Data Collection Plan: Identify the types of images needed and where they will be sourced from.
- Choose the Right Annotation Strategy: Determine the most effective annotation methods based on project goals.
- Incorporate Feedback Loops: Regularly evaluate the annotation output to improve quality and consistency.
- Test and Iterate: Run tests with your classification models and optimize based on performance metrics.
Challenges in Working with Image Datasets
While leveraging image datasets for classification presents numerous opportunities, businesses may encounter challenges in the process. Here are a few common ones:
Common Challenges
- Data Quality Issues: Poorly annotated data can lead to inaccurate model predictions.
- Scalability Problems: Managing large volumes of images can strain resources without a proper annotation strategy.
- Resource Allocation: Finding skilled annotators can be time-consuming and costly.
- Continuous Maintenance: Models need regular updates as new data emerges to remain relevant.
The Future of Image Classification in Business
The rise of AI and machine learning technologies will undoubtedly shape the landscape of image datasets for classification. As businesses continue to adopt these technologies, the demand for high-quality annotated datasets will increase. Here are some trends to watch:
Emerging Trends
- Automation of Data Annotation: The integration of AI in annotation tools will streamline processes and enhance quality.
- Real-Time Data Processing: Businesses will increasingly require systems that can process and annotate data in real time.
- Expansion of Industry Applications: Sectors such as healthcare, retail, and automotive will utilize image classification more extensively for various applications.
- Focus on Ethical AI: As image classification becomes ubiquitous, ethical considerations around bias and transparency will take center stage.
Conclusion
In conclusion, the ability to effectively utilize image datasets for classification can propel businesses toward unprecedented heights. By investing in the right data annotation tools and platforms, organizations not only optimize their operations but also enhance their decision-making, improve customer satisfaction, and achieve a substantial return on investment. As we advance towards a future driven by AI, the need for excellence in data handling will become even more critical. Don't miss out on the opportunity; embrace the power of data today with KeyLabs.ai.